AI-Powered Customer Support Automation
Reducing support ticket volume by 35% through ML-based classification
Key Result 1
35% reduction in support tickets requiring human intervention
Key Result 2
28% faster resolution time for all tickets
Key Result 3
42% improvement in first-response time
Project Context
Industry
Technology & SaaS
Problem Domain
Customer Experience & Support
My Contribution
Requirements, ML Solution Design, Implementation Lead
Technologies & Skills
Overview
The customer support team was overwhelmed with a high volume of tickets, many of which were repetitive and could be automated. I led the initiative to implement an AI-powered system to classify, route, and automatically respond to common support queries.
The Challenge
The support team was handling over 10,000 tickets monthly, with response times averaging 24+ hours. Customer satisfaction was declining, and support costs were rising. The team needed a solution to handle the increasing volume without sacrificing quality.
My Approach
- 1
Conducted extensive analysis of historical support tickets to identify patterns and common issues
- 2
Collaborated with data scientists to develop and train ML models for ticket classification
- 3
Worked with engineering to integrate the ML system with existing support infrastructure
- 4
Implemented a phased rollout approach to minimize disruption and gather feedback
- 5
Established clear metrics to measure success and ROI
The Solution
We developed an ML-based system that could automatically classify incoming support tickets, route them to the appropriate team, and provide automated responses for common issues. The system used natural language processing to understand the intent behind customer queries and improved over time through continuous learning.
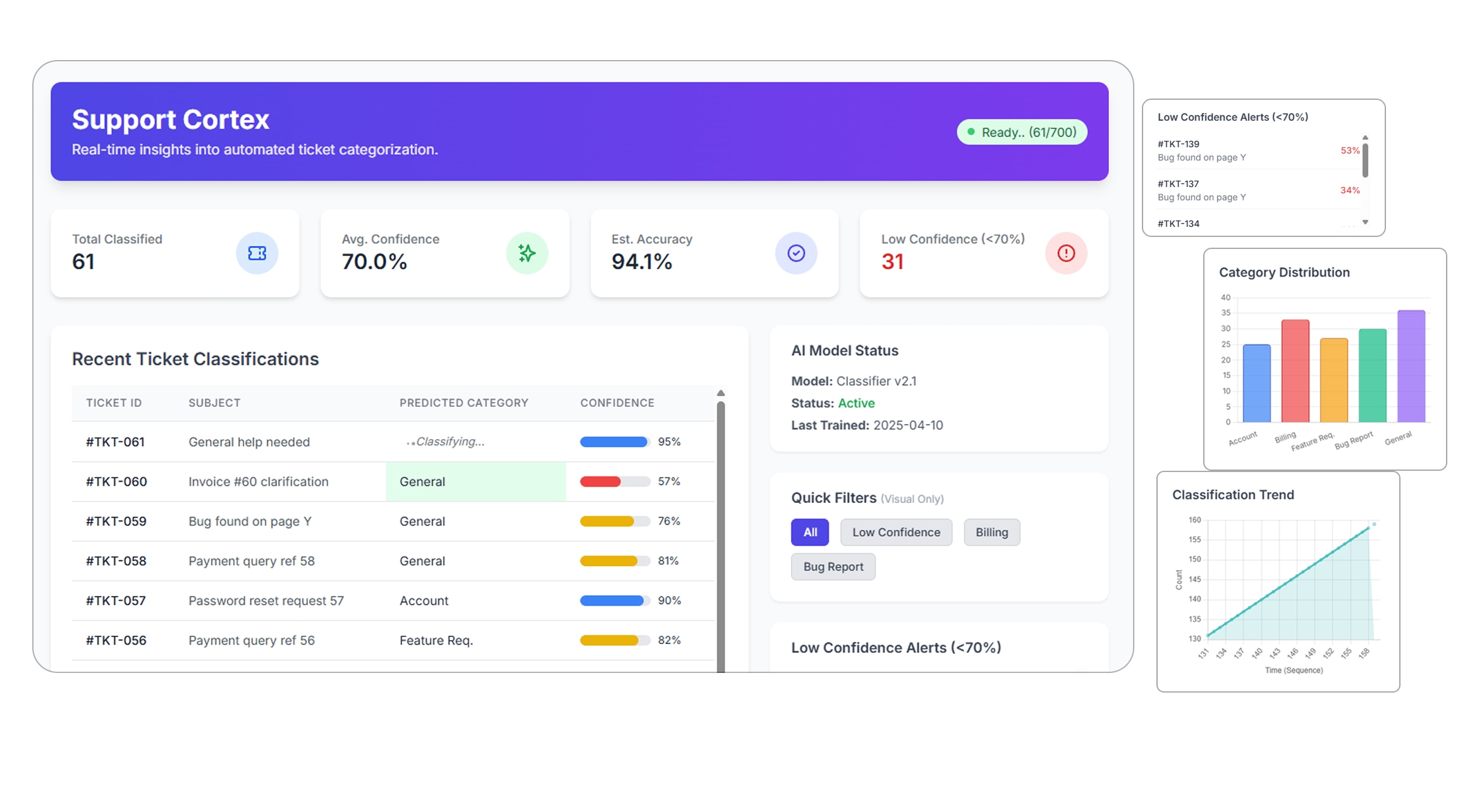
The ML classification dashboard showing real-time ticket processing
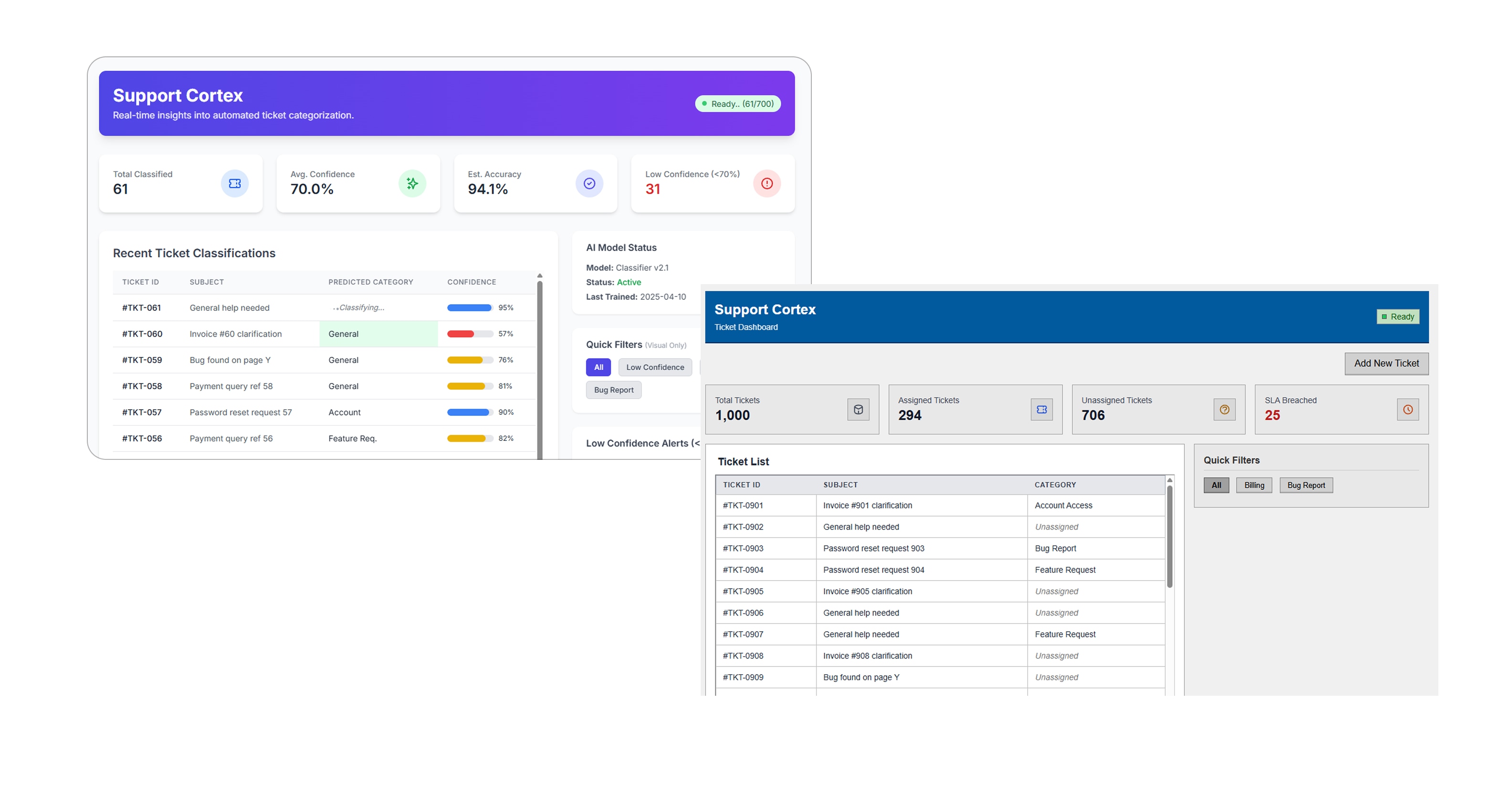
Comparison of key support metrics before and after implementation
Results & Impact
35% reduction in support tickets requiring human intervention
28% faster resolution time for all tickets
42% improvement in first-response time
18% increase in customer satisfaction scores
$450K annual cost savings through improved efficiency
"The AI support system has transformed how our team operates. We're now able to focus on complex issues while the system handles routine queries, resulting in faster response times and happier customers."
Director of Customer Support
SaaS Technology Company
Key Learnings
The project highlighted the importance of starting with a focused scope and expanding gradually. Initially, we tried to automate too many ticket types, which reduced accuracy. By focusing on the most common 20% of issues first, we achieved better results and built confidence in the system before expanding.